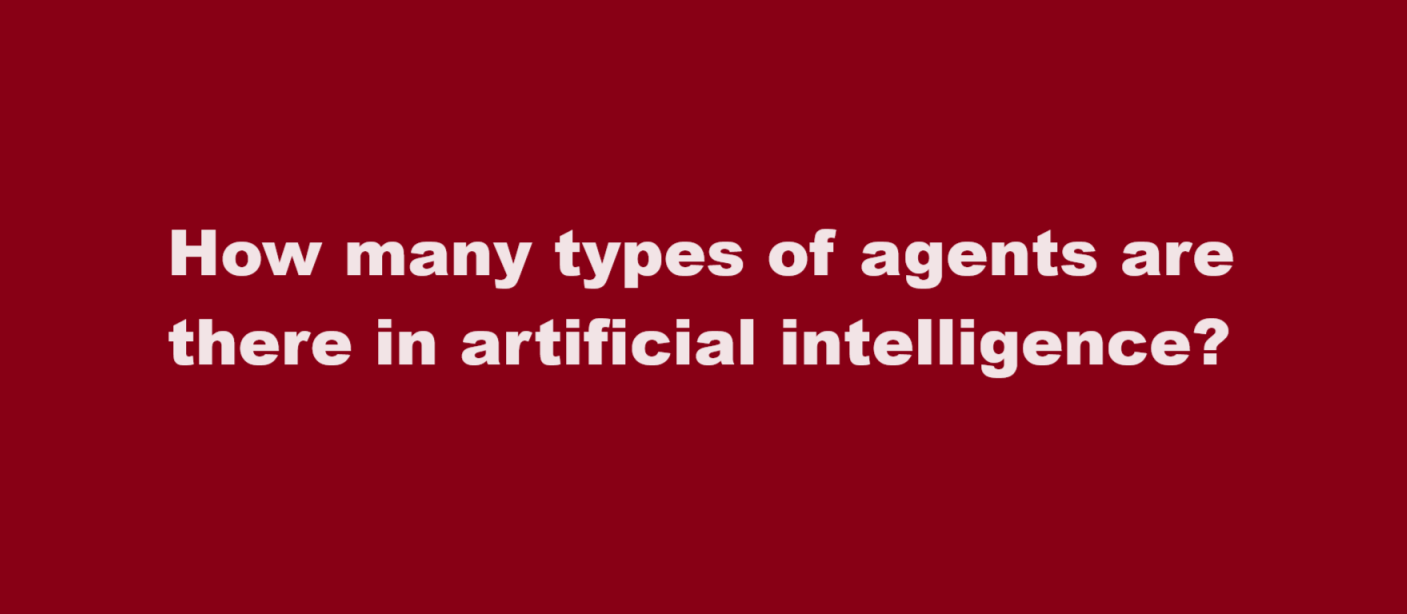
Artificial Intelligence (AI) is rapidly evolving and changing how we interact with technology. AI consists of various types of agents designed to efficiently complete tasks in various settings. In this article, we will explore how many types of agents there are in AI and what roles they play. We’ll also discuss how AI agents can affect our lives, from robot vacuum cleaners to autonomous cars.
Artificial Intelligence Agents
Artificial Intelligence (AI) is a rapidly growing field that promises to revolutionize how we interact and work with machines. One of the most exciting aspects of AI is its ability to create agents or programs designed to act independently and make decisions without direct human supervision. The type and number of agents vary depending on the application, but several common types are used in Artificial Intelligence.
Types of AI Agents
AI agents are categorized into two primary groups: reactive and deliberative. Reactive agents respond to their environment without relying on experience or memories. An example of this type of agent would be self-driving cars that utilize sensors to interact with their environment. On the other hand, deliberative agents can make decisions based on learned experiences or stored memories. These AI systems can interpret data from previous situations to make better decisions in future scenarios.
B-tech artificial intelligence and data science colleges in Tamilnadu
B-tech artificial intelligence and data science colleges in Tamilnadu are becoming increasingly popular as the demand for professionals in these fields grows. As AI and data science become increasingly prominent in the tech industry, college students are keen to gain knowledge in these areas. With the rise of technology giants like Google, Amazon, Microsoft, and IBM investing heavily in AI and Machine Learning research, there is a great need for trained professionals with expertise in these fields.
Many B-tech Artificial Intelligence (AI) and Data Science Colleges spread across Tamilnadu offer excellent opportunities to learn from experienced teachers who specialize in this field. Each college provides various courses related to AI, such as Natural Language Processing (NLP), Computer Vision (CV), Robotics, Deep Learning & Neural Networks, etc., and Data Science which covers topics such as Big Data Analytics, Data Mining & Warehousing, etc.
Reactive Machines
Reactive Machines, or agents in artificial intelligence (AI), are systems designed to interact with their environment. They are called reactive because they do not rely on memories or experiences; instead, they make decisions based solely on their current state and what is happening within their immediate environment. AI agents can be divided into two primary categories: symbolic systems and sub-symbolic systems.
Symbolic systems use abstract symbols to process data and interpret the world around them. This type of agent is based on logic, rules, and language processing, allowing it to create plans for completing tasks as efficiently as possible. Sub-symbolic systems, also known as connectionist machines, use neural networks to analyze data and draw conclusions from patterns. These agents work similarly to humans’ learning by connecting similar data points over time.
Limited Memory Agents
Limited memory agents can store and access previously encountered data to make informed decisions about future actions. This type of agent is equipped with an internal model that stores relevant information about its environment, such as past events, recent observations, current goals, etc., allowing it to make intelligent predictions based on what it has learned from its experience.
Goal-based Agents
Goal-based agents are a type of Artificial Intelligence (AI) agent that relies heavily on the presence of a goal. They are typically used to complete tasks requiring a specific action or output to succeed. Goal-based agents use heuristics or learning methods, such as reinforcement learning, to find the best way to achieve their objectives. It is done by recognizing patterns in data and using problem-solving techniques or logical reasoning to develop an action plan for reaching the desired outcome. The ability of goal-based agents to recognize patterns and make decisions based on this analysis makes them incredibly valuable for solving complex problems. In addition, they can be programmed with different levels of autonomy to adjust their strategies depending on the complexity of the task.
Utility-based Agents
Utility-based agents are known as goal-based or rational agents, whose main aim is to maximize rewards and minimize costs to meet goals. These agents use Markov Decision Processes (MDPs) and Reinforcement Learning (RL) to assess situations and take actions for maximum gain. They are designed to recognize patterns in data over time so that they can make informed decisions with little or no human input. Utility-based agents have been used successfully in many fields, including robotics, finance, gaming, text processing, and autonomous vehicles.
Learning Agents – Artificial intelligence poster
In this poster, readers will be introduced to supervised learning agents, unsupervised learning agents, reinforcement learning agents, and their subsets, such as recurrent neural networks and deep reinforcement learning. Supervised Learning Agents use labeled data sets to create training algorithms that enable machines to recognize patterns in new data points.
Unsupervised Learning Agents use unlabeled data sets to detect correlations between variables and reveal hidden patterns or structures within the data set. Reinforcement Learning Agents use rewards-based approaches to teach machines how to act in certain situations or environments.
Final Verdict
How many types of agents are there in artificial intelligence? Is there a wide variety of agents available for use in AI technology? Depending on the exact application and industry of use, the number and type of agents used can vary greatly.
There are reactive agents who respond to input stimuli directly without any pre-programmed behavior; goal-based agents with a specific task or objective they must reach; utility-based agents who make decisions based on an assigned “utility” measure; and learning agents that improve their performance over time with experience.
For example, an autonomous car requires reactive and goal-based agent technology. The reactive agent would detect objects in its path, such as other cars or pedestrians, while the goal-based agent would dictate how it should react to those objects.
Read Also : How to detect fake news detection using machine learning